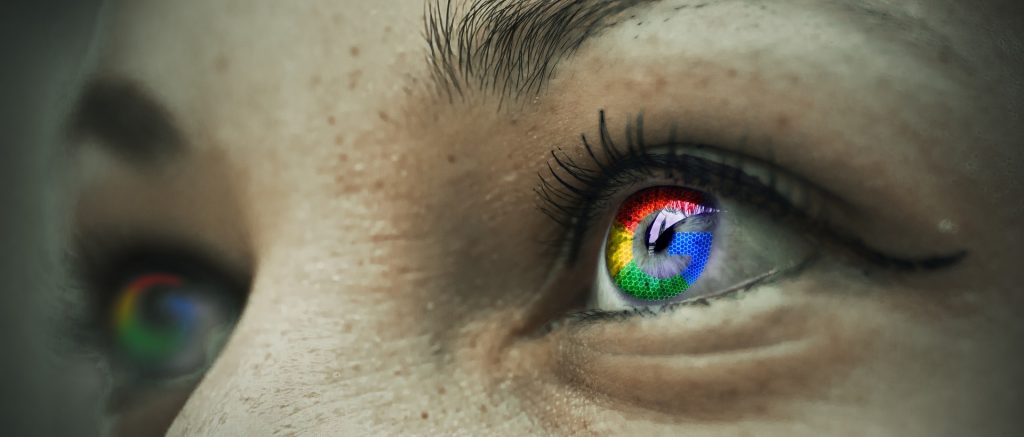
Machine learning (ML) has left a lasting impression in many business areas and tech companies have been benefited the most from it. Google, world’s top tech giant has been leading ML research from the very beginning. Its core business, which includes online advertising as well as cloud computing, is making the most out of ML. Now, Google’s powerful search engine is also powered by ML algorithms for better optimisation of websites (abbreviated SEO for ‘search engine optimisation’).
With the growing number of internet traffic, Google has switched to a more sophisticated and structured SEO. While most challenges have been overcome, we shall here discuss how a machine learning approach to the already established ML algorithms in Google’s SEO might help reduce complications in the search engine.
The Slow Rise Of ML In SEO
Earlier, computer algorithms for SEO incorporated changes as and when they were observed, and made improvements in it. These improvements were bundled out as an update for the algorithms, and this sometimes meant abrupt changes at one go. Google usually updates their SEO algorithms frequently, and with every update in SEO, the rankings and search engine results page (SERP) are affected, either positively (more reach/traffic) or negatively (less reach/traffic). This is important because businesses that depend on their websites suffer as a consequence of these SEO changes.
In the recent years, Google started adopting ML for SEO. For example, in 2015, Google brought out RankBrain, an ML algorithm that analyses and processes search results which was a part of Google’s ranking algorithm. This has changed SEO significantly, since now it has moved beyond that analysing keywords and links. SEO algorithms no longer follow the traditional indexing. After Google’s restructuring of SEO with Caffeine, indexing of search results became more efficient than ever, with fresher content given high priority.
But, this came with an innate problem related to algorithm updates. Although Caffeine provided more transparency in sorting out ranking factors, it required human intervention to oversee ranking changes in line with the algorithm updates. RankBrain helped overcome this problem, as it analysed large parts of search results’ data by providing ranking signals in addition to ranking factors.
Marcus Tober, founder and CTO at Searchmetrics, a popular search engine analytics platform that focuses SEO on a global level, says that RankBrain merges hundreds of ranking factors considered by Google, into one. These factors are prioritised differently for each query. With ML in RankBrain, experts at Google could now focus on polishing user content without having to worry about algorithmic complexity.
Predicting The Ranking And Algorithms
Now comes the elephant in the room which is using machine learning on these algorithms themselves. This means predicting the SEO rankings and algorithms beforehand with the help of ML. A number of SEO tools are available in the market to collaborate with Google’s SEO to provide custom search engine solutions. These tools predict algorithmic changes quickly in addition to sorting ranking factors efficiently.
Building a machine learning model for Google SEO is a formidable task. Apart from providing the best search results based on the content, the ML model should also look out for eliminating security vulnerabilities such as spam and phishing, and other threats which are mostly generated through emails. For this, ML requires an influx of huge data obtained and assessed by human experts. Therefore, it needs to analyse the good and bad sides of SEO constantly with and with precise efficacy.
Another challenge is to go through algorithmic changes based on keyword. Google’s algorithm responds differently to different keywords. As mentioned earlier, SEO tools can help with this problem as well. These tools can analyse and categorise keywords catered to individual businesses in the search engine environment. This also poses instances of same keywords for businesses with their rivals. Jason DeMers, CEO of content marketing agency AudienceBloom elaborates this downside perfectly. He says, “On the flip side, if a brand has to worry about many different keywords that span various competitors in each environment, then investment in these newer technologies may be warranted. SEO teams need to keep in mind that they can’t simply apply what they’ve learned in one keyword environment to another. Some sort of adaptive analysis must be used.”
Conclusion
As new advances take place in machine learning, it is important to take a note if they are making the right impact. In areas such as SEO, it requires rules set by experts before ML projects are deployed in action. In addition to that, newer tools which possess AI and ML features can also be integrated with SEO and should be implemented with utmost care. Since today is the golden era of machine learning and artificial intelligence, SEO can present a different digital world altogether.
[“Source-analyticsindiamag”]